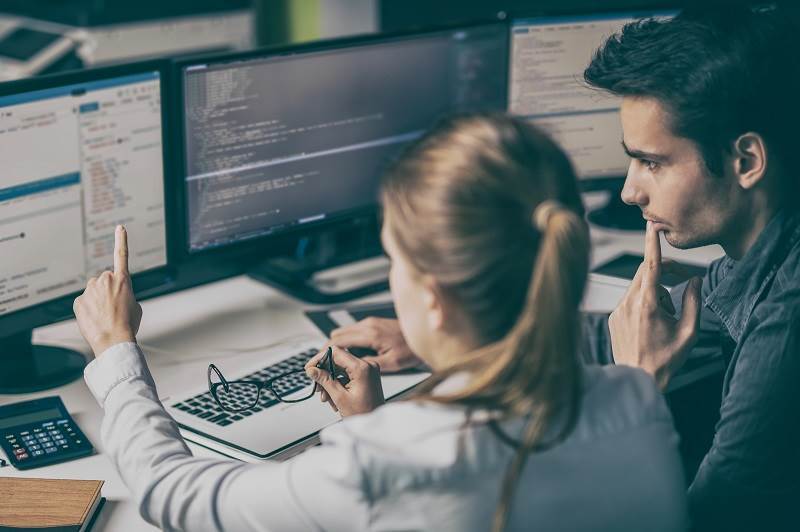.jpg)
By Steve Cavolick
If you are fortunate enough to have mastered the organization and quality of your data to support operational reporting, you will no doubt be looking for new ways to monetize the data you have. Predictive and prescriptive analytics is the next step to maximizing the value of those assets so you can improve the top or bottom line.
The market for predictive analytics platforms comes down to commercially available platforms that you can purchase and open source solutions. So how do you know which one is best for you? Let’s look at some options and their advantages in the following paragraphs.
For open source, the two major options are R and Python. Until 2017, R had been the more popular platform, but Python overtook it in early 2017. The graph below from Google trends shows how quickly Python has grown in the data scientist community. The red line represents R and the blue line describes Python.
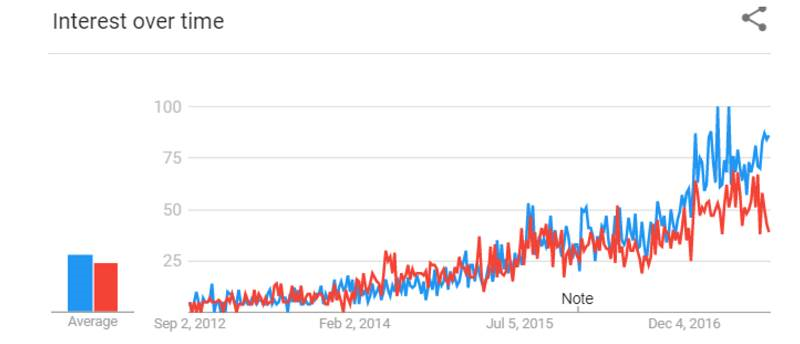
Both are programming languages, and depending on who you ask, one is easier to pick up than the other. The key to their awesome flexibility is libraries of thousands of functions, giving you a virtually limitless set of algorithms to create applications that can answer almost any question. While the flexibility within these platforms is great, there are issues with these tools (especially R) that developers must contend with: processing speed, connectivity to data sources, and collaboration on models. In addition, while there is a giant community of developers who can help you with questions, support can be an issue for those who need more hand-holding.
For off-the-shelf software, SAS and SPSS are the heavyweights in this arena. Both are mature platforms (in use since the 1960s) that provide predictive models and advanced analytics to help solve business problems. They offer both graphical interfaces with drag-and-drop, and scripting for development. Besides GUI development environments, the reason they are popular is that they offer capabilities where some of the open source platforms fall short: scalability and collaboration for multiple developers, connectivity to many types of data sources, and more options for visualizing output from predictive models. Plus, they both sport traditional support mechanisms across multiple channels for when assistance is required.
So which approach is best for you? We can’t tell you which approach is best for your organization and your developers. What we can tell you is that the products are not mutually exclusive. Many of our customers have some people who build models in one of the open source tools and some who use SAS or SPSS.
And there is value in that approach: open source users who also use SAS or SPSS gain data management capabilities, output management (although the open source solutions have come a long way in visualizations), and a perfect way to share packages to a wider audience, including non-R/Python users. Plus, SAS and SPSS users get access to the massive amount of statistical functions found in open source, allowing them to perform even more sophisticated analyses.
Using open source predictive packages with mature off-the-shelf platforms has many advantages and is definitely something you should consider.
The LRS Big Data and Analytics team has 20 years of experience in analytics and information governance, including predictive and prescriptive analytics with the tools and platforms mentioned above. If you are interested in understanding how we can help you create a competitive advantage with predictive applications, please fill out the form below and let us know how we can help.
About the author
Steve Cavolick is a Senior Solution Architect with LRS IT Solutions. With over 20 years of experience in enterprise business analytics and information management, Steve is 100% focused on helping customers find value in their data to drive better business outcomes. Using technologies from best-of-breed vendors, he has created solutions for the retail, telco, manufacturing, distribution, financial services, gaming, and insurance industries.