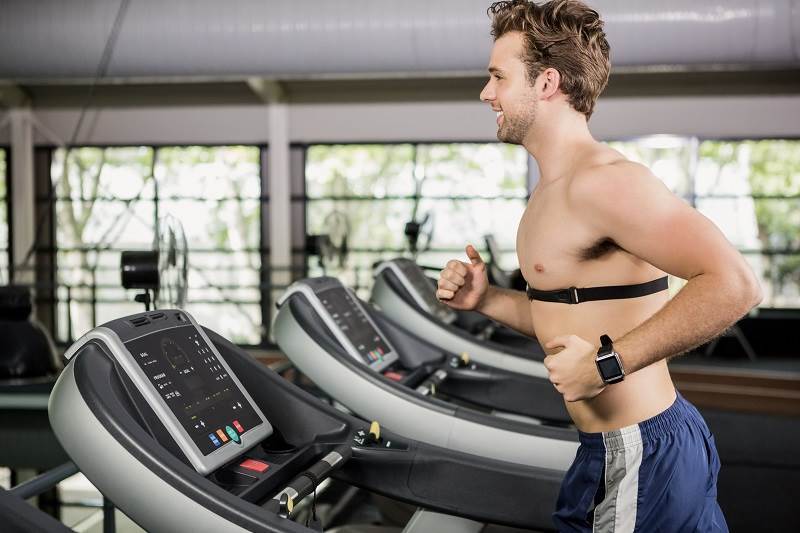
By Steve Cavolick
With Artificial Intelligence, Machine Learning, and Natural Language Query stealing the spotlight on the analytics hype curve right now, it is easy to forget about the styles of analytics that have been in use since before people ever imagined machines could behave like humans.
One such area of analytics is statistics. If you have forgotten most of what you learned in your Stats 101 class, statistics is a collection of tools and models that make it easier to interpret and understand the meaning of qualitative data. Statistics allows you to compare data sets and determine how alike they are to each other, where outliers exist, and illustrates defining characteristics of the data.
A key concept in statistics is correlation, which explains how strongly two factors are related in a given data set. Correlation is usually visualized with a scatter plot, where one factor is plotted on the X-axis and one factor is plotted on the Y-axis. If the factors are not closely related, the data points exist in the shape of loosely defined cloud; the stronger the correlation of the factors, the more the data points resemble a straight line. If the correlation is positive (the more time spent exercising and calories burned, for example) the line slopes upward. If the correlation is negative (time spent playing video games and grades), the line slopes downward. Strength of correlations are expressed on a scale of +1 to -1, where 0 represents no correlation and 1 or -1 is perfect correlation.
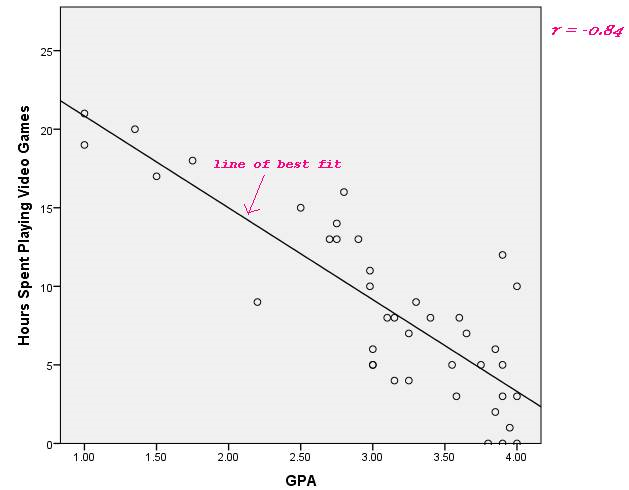
Example of a strong negative correlation. R represents correlation strength.
But does statistics still have a place in modern analytics? What kind of problems can be solved with statistics? Most weekend and professional athletes are now instrumented with wrist-based monitors that display your heart rate. There is growing distrust among athletes of the readings supplied by these monitors. In fact, several studies used statistics to illustrate that wrist-based heart monitors are not the way to go if you need the most accurate measurements.
The Journal of The American Medical Association Cardiology performed a study that compared the accuracy of chest strap heart monitors and those worn on the wrist. The study concluded that chest straps had a 0.99 correlation with electrocardiograph readings during exercise, but that wrist monitors varied between 0.83 and 0.91 correlation. Another study in the Medicine & Science In Sports & Exercise Journal found that the correlation between wrist heart monitors and actual heart rate varied even more, from 0.75 to 0.92, depending on the manufacturer.
An additional test executed by BMC Sports Science, Medicine, and Rehabilitation found that the heartbeat numbers provided by wrist-based monitors during running were within 10 beats per minute 95% of the time, and that the average error was 3 beats per minute. The conclusion here is that for a one hour run, there will be a couple of minutes where the heart rate monitor provides a number that is 10+ beats off. You may think that these readings are “close enough,” and still acceptable, but for athletes who depend on monitoring the heart rate as a way to stay in certain aerobic zones, inaccurate readings could lead to lack of recovery during training or worse, hitting the wall during a race.
As seen with the example above, statistics still has a valid place in your enterprise for solving problems. If you are new to advanced analytics, think of statistics as a gateway to predictive analytics and artificial intelligence and helping you progress your journey along the analytics maturity curve.
The LRS Big Data and Analytics team has over 20 years of experience in statistics, information management, predictive analytics, and data warehousing. If you are interested in understanding how we can help you find value in your data with advanced analytics, please fill out the form below to request a meeting.
About the author
Steve Cavolick is a Senior Solution Architect with LRS IT Solutions. With over 20 years of experience in enterprise business analytics and information management, Steve is 100% focused on helping customers find value in their data to drive better business outcomes. Using technologies from best-of-breed vendors, he has created solutions for the retail, telco, manufacturing, distribution, financial services, gaming, and insurance industries.